The AI-energy paradox: Will AI spark a green energy revolution or deepen the global energy crisis? — Part 1
Artificial intelligence (AI) is expanding at breakneck speed, presenting a paradox for global energy systems. On one hand, AI-driven innovations promise efficiency gains in renewable energy management and smarter grids. On the other, the surging power demands of AI threaten to strain electricity infrastructure and increase reliance on fossil fuels.
Current projections indicate data centres — the digital fortresses powering AI — could consume over 1,000 TWh of electricity by 2026, roughly double their 2022 usage. (For perspective, that’s comparable to Japan’s annual power consumption, or about 90 million US homes.)
In the European Union alone, data centre energy use is forecast to reach 150 TWh by 2026, ~ four per cent of EU demand. Gartner even predicts that 40 per cent of existing AI data centres will hit power capacity limits by 2027, underscoring the urgent infrastructure challenge.
This surge places immense pressure on power grids. Cutting-edge AI models require enormous energy: Training a single large language model (LLM) like OpenAI’s GPT series can devour tens of gigawatt-hours of electricity . Some hyper-scale AI data centres already draw 30-100 megawatts each, and future facilities may exceed 1,000 MW (1 gigawatt) — about the output of a large power plant .
One industry analysis notes tech giants are pursuing “gigawatt-scale” data centre campuses to support AI workloads . By 2030, Microsoft and OpenAI’s planned “Stargate” supercomputer could require an astonishing five GW of power.
In response, tech companies are exploring diverse energy strategies. Google, for instance, is investing in advanced nuclear power: it signed a deal to purchase energy from small modular reactors (SMRs), aiming to add 500 MW of carbon-free power by 2030.
Microsoft is turning to nuclear with the Three Mile Island nuclear power plant deal, Amazon, and Meta are turning to conventional power plants — in some regions, new natural gas-fired generators — to guarantee reliable juice for AI data centres, a strategy supported by utilities. In Wisconsin, regulators approved a US$2 billion gas plant deemed “critical” for Microsoft’s new AI hub.
These moves underline a hard truth: renewables alone can’t yet meet AI’s ravenous base-load demand, prompting a dual-track energy race between carbon-free solutions and fossil fuels.
This brings up pressing questions for business leaders:
- Will AI ultimately drive sustainability gains or an energy crisis?
- How are regional disparities and geopolitics shaping AI’s energy footprint?
- What technological breakthroughs could enable sustainable AI growth?
- And how should corporate strategy adjust to balance AI’s benefits against its energy and carbon costs?
This three-part guide examines the forces at play — from data centre trends and energy innovations to policy and geopolitical factors — to help corporate decision-makers navigate AI’s energy revolution.
The goal: understand the macro and geopolitical impacts of AI’s energy consumption, and chart a course that leverages AI’s power responsibly and sustainably.
The energy cost of AI: Hard truths and hidden opportunities
Global data centre electricity consumption reached an estimated 460 TWh in 2022, with AI and cryptocurrency operations accounting for roughly 14 per cent of that load, according to the International Energy Agency (IEA).
Now AI is pushing those numbers dramatically higher. Projections show data centres worldwide could consume over 1,000 TWh by 2026 — roughly doubling in just four years. By 2030, some forecasts see a further 160 per cent increase in data centre power demand driven by AI.
Also Read: Eco-investing: Driving change through climate technology and strategic finance
This growth is concentrated in key AI hubs and “cloud clusters” with serious consequences for local grids:
- In Northern Virginia’s famed “Data Centre Alley,” the massive concentration of servers has led to power quality issues. The region now experiences voltage distortions four times higher than the US average, raising the risk of appliance damage and even fires for surrounding communities. Utilities warn that traditional grid infrastructure is straining to keep up with the load.
- In central Ohio, data centre capacity has quadrupled since 2023, consuming so much electricity that utility AEP had to halt new data centre connections, despite a 30 GW queue of projects waiting to plug in. Simply put, the grid can’t be expanded fast enough to accommodate the sudden surge in demand.
- Ireland faces a similar crunch — by 2026, data centres are projected to gobble up 32 per cent of Ireland’s electricity. Dublin’s metro grid is so stressed that the government imposed a moratorium on new data centres in the area, shifting over US$4 billion in planned investments to other countries.
The energy intensity of AI is a key reason demand is outpacing capacity. A few eye-opening facts illustrate the scale:
- Training a single large AI model can consume enormous amounts of electricity. For example, training ChatGPT/GPT-3 (with 175 billion parameters) is estimated to use on the order of 1-1.3 GWh (gigawatt-hours) of energy — roughly the yearly electricity usage of over 1,000 US homes. And that’s for one training run. Newer models like GPT-4 are even more power-hungry — estimates suggest on the order of 50-60 GWh for a full training cycle, which would be enough to power ~4,500 homes for a year (and emits tens of thousands of tons of CO₂). In other words, one large AI model’s training = years of household electricity.
- Running AI models (inference) is also energy intensive. AI queries consume about 10× more electricity than a typical Google search. Every time you ask ChatGPT a question, a network of GPUs fires up, drawing far more power than a standard web search. Multiply this by millions of queries, and the energy adds up fast. Microsoft and Amazon have responded by securing huge dedicated power supplies for their cloud AI operations — on the order of 500 MW to 1,000 MW per data centre campus — to ensure they can handle the surging demand. For perspective, a single 1,000 MW data centre campus could consume as much power as 750,000 homes.
- The sheer consumption of top tech companies is staggering. In 2023, Microsoft and Google each used ~24 TWh of electricity — more power than entire countries like Iceland, Jordan, or Ghana consume in a year. This puts their usage above that of over 100 nations. While these firms have aggressive renewable energy programs, the scale of their energy draw highlights how big the AI computation boom has become.
- The cloud giants are investing heavily to keep this sustainable. Microsoft recently announced a US$10+ billion deal with Brookfield to develop 10.5 GW of new solar and wind farms by 2030 — an unprecedented corporate clean power purchase aimed squarely at running its AI and cloud data centres on carbon-free energy. Amazon and Google are similarly pouring funds into renewables and even experimental technologies (like advanced geothermal and batteries) to offset their growing AI footprint.
Despite these efforts, power constraints are emerging as a growth limiter for AI. Industry analysts warn that in the next few years, many data centre operators (especially those not backed by big tech) may find it difficult or prohibitively expensive to get the electricity they need.
Gartner projects that by 2027, 4 in 10 AI data centres worldwide could hit their power capacity ceiling, meaning their expansion will be stalled by energy shortages. For enterprises, this could translate to slower cloud rollouts or higher costs as energy prices rise.
However, within this hard truth lies a hidden opportunity — AI itself can help solve the energy challenge. As we’ll explore, the same technology driving up consumption can also drive greater efficiency and new solutions, if wielded wisely.
Also Read: The key to tackling climate change: Electrify shipping
Comparing AI models: Power hunger from GPT to KNN
Not all AI is equally power-hungry. There is a vast gap in energy consumption between large, state-of-the-art AI models and more traditional algorithms. Understanding this spread can help leaders choose the right AI tools for the job — balancing capability and cost. The table below compares examples of AI models:
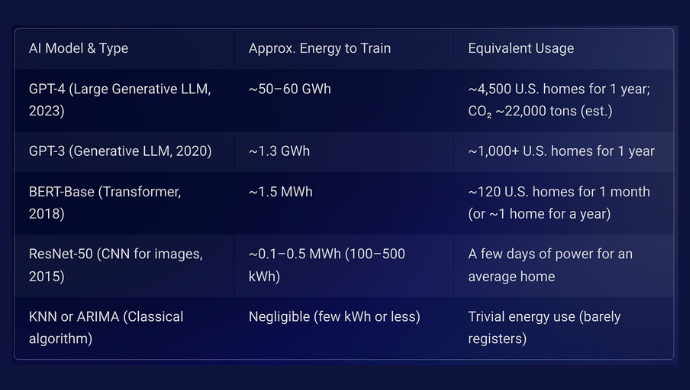
Table: Energy requirements for training various AI models range over orders of magnitude. Cutting-edge deep learning models (top rows) consume enormously more energy than smaller neural nets or classical machine learning methods (bottom rows). Choosing a right-sized model can avoid wasting power.
As the table shows, today’s largest AI models (like GPT-3/4) dwarf earlier AI in power needs. Training GPT-4 can use about 50,000× more energy than training a typical convolutional neural network (CNN) like ResNet-50 used for image recognition.
And an old-school algorithm like k-nearest neighbors (KNN) or an ARIMA forecast model might use a million-times less energy — essentially negligible in comparison.
This doesn’t mean companies should avoid large AI models altogether; rather, it underscores the importance of right-sizing AI to the task. You don’t always need a billion-parameter model if a simpler one works — and the energy (and cost) savings from a leaner approach can be huge.
Key takeaway: AI’s energy footprint isn’t uniform. Generative AI and other complex models can be incredible but come with extreme energy costs.
Business leaders should evaluate whether a smaller, more efficient model could meet their needs. In many cases, optimized or “distilled” models, or running AI at the network edge, can deliver acceptable performance while using a fraction of the power. This efficiency-centric approach to AI adoption will become increasingly vital as energy pressures mount.
Fossil fuel lock-in vs a nuclear renaissance
The tug-of-war between AI’s energy demand and clean energy supply is pushing companies down two very different paths. On one side, some firms and regions are doubling down on fossil fuels to keep the lights on for AI. On the other, there’s a growing movement toward a nuclear revival (along with renewables) to power AI sustainably.
Also Read: What does Trump mean for SEA climate scene?
On the fossil fuel front, oil and gas producers see AI’s rise as a new source of demand for hydrocarbons. BP’s CEO Murray Auchincloss, for example, predicts AI’s infrastructure build-out could drive an extra 3-5 million barrels per day of oil demand growth through the 2030s, as data centres and associated supply chains consume more energy (fuel for generators, diesel for construction, etc.). Likewise, Shell’s latest Energy Security Scenarios project natural gas demand reaching 4,640 billion cubic meters annually by 2040, partly to fuel backup generators for data centres and provide grid stability in an AI-enabled economy.
These trends raise concerns that AI could inadvertently lock in a new wave of fossil fuel dependence right when the world is trying to decarbonise. For instance, in the US, some utilities are proposing 20+ GW of new gas-fired power plants by 2040largely to meet data centre growth.
This runs directly against climate goals — building gas infrastructure that could last 40-50 years to serve what might be a short-term spike in AI-related demand.
Conversely, a potential “nuclear renaissance” is being driven by AI’s 24/7 power needs and corporate clean energy pledges. Nuclear power offers steady, carbon-free electricity that is highly appealing for always-on AI workloads. We’re seeing concrete steps in this direction:
- Microsoft is investing US$1.6 billion to help reopen the dormant Three Mile Island nuclear plant in Pennsylvania, aiming to secure 24/7 carbon-free power for its AI data centres by 2028. This would repurpose an existing nuclear reactor to directly feed Microsoft’s cloud operations — a bold bet on nuclear as a reliable green energy source for AI.
- Amazon and Google have each committed at least US$500 million in financing to startup companies developing small modular reactors (SMRs). Their goal is to have about 5 GW of new nuclear capacity from SMRs online by the mid-2030s. Google’s agreement with Kairos Power, for instance, targets the first SMR operational by 2030. If successful, these would be game-changers: modular reactors could be built near data centres to provide dedicated clean power.
- In Europe, policymakers are increasingly viewing nuclear as essential for meeting AI’s power demands. The EU projects that nuclear-powered data centres (where data centres are co-located with nuclear plants or dedicated reactors) could supply 15-25 per cent of the new electricity needed for AI and digital growth through 2030. France and the UK have floated incentives for data centre operators to hook into existing nuclear plants, while countries like Romania and Estonia are partnering on SMR deployment with an eye toward tech sector needs.
The contrast is striking: Will the AI era deepen our fossil fuel dependence or accelerate the shift to alternative energy?
In practice, both are happening — but the balance could tip one way or the other based on economics and policy. Natural gas plants currently often win on cost and speed (a gas turbine can be built faster than a nuclear plant and is a proven solution to instantly boost capacity).
Indeed, “the only concrete plans I’m seeing are natural gas plants,” notes one energy consultant about data centre expansions. Yet, as carbon costs rise and modular nuclear tech matures, nuclear and renewables could prove the more attractive long-term play.
For corporate leaders, this means energy strategy is becoming inseparable from AI strategy. Companies may need to directly invest in energy projects (like Microsoft’s and Google’s deals) to ensure their AI ambitions have a viable power supply. Those that succeed in securing reliable, clean energy will not only meet sustainability goals but also gain an operational advantage (avoiding the risk of power constraints slowing their AI deployments).
This is part one of a three-part series exploring AI’s energy impact.
Part two of this series examines how AI can enhance energy efficiency and optimise grid management to address this challenge.
This article was originally published here and co-authored by Xavier Greco, Founder and CEO of ENSSO.
—
Editor’s note: e27 aims to foster thought leadership by publishing views from the community. Share your opinion by submitting an article, video, podcast, or infographic.
Join us on Instagram, Facebook, X, and LinkedIn to stay connected.
Image courtesy: DALL-E
The post The AI-energy paradox: Will AI spark a green energy revolution or deepen the global energy crisis? — Part 1 appeared first on e27.